Ted Ding
Autonomous vehicles and robots are required to operate safely not only in clear weather, but also in the presence of fog when visibility is poor. On a foggy day, water droplets suspended in the atmosphere interact with light and thus degrade the quality of data acquired by most automotive sensors. Normal machine vision algorithms, which are predominantly developed for operation in clear weather, struggle or even completely fail under low visibility conditions.
In this research project, two interlinked, stereo camera-based systems are designed, implemented and validated with the aim of advancing robust visual perception in fog. Firstly, given a sequence of stereo foggy images, a methodology which estimates the parameters of a fog model and dynamically updates them is developed. Knowledge of these parameters not only enables characterisation of the nature of the fog, but also provides key information as input to the second system. The capability of the proposed method is fully demonstrated from various aspects, including producing very accurate estimates and responding adaptively to spatially variant fog. Secondly, given a single pair of stereo foggy images with known fog parameters, an algorithm which simultaneously performs dense stereo reconstruction and image defogging is presented. Dense depth data can benefit downstream, high-level vision tasks and defogged images are useful for human drivers to better understand the scene. Jointly tackling these two problems proves to be a more effective approach than dealing with them separately. This is facilitated by knowledge of the fog parameters which can be reliably estimated using the first system.
In addition, a dataset comprising sequences of real, high-quality stereo foggy images under a variety of visibility conditions is introduced. Moreover, camera calibration is performed in a controlled laboratory to determine the photometric parameters, knowledge of which is a prerequisite for correctly applying the fog model. This first-of-its-kind dataset is extensively used for the development and evaluation of the two systems above. It is released to the public to address the concern about a lack of autonomous driving datasets which focus on foggy scenes.
Publications:
- K. Zhang, Y. Ding, S. Xu, Z. Hong, X. Kong, S. Wang, "CURL-MAP: Continuous Mapping and Positioning with CURL Representation†", in 2024 IEEE International Conference on Robotics and Automation (ICRA).
- Y. Ding, A. M. Wallace, S. Wang, "Estimating Fog Parameters from an Image Sequence using Non-linear Optimisation", in 2024 IEEE/CVF Winter Conference on Applications of Computer Vision (WACV).
- Y. Ding, A. M. Wallace, S. Wang, "Variational Simultaneous Stereo Matching and Defogging in Low Visibility", in 2022 British Machine Vision Conference (BMVC).
My name is Yining (Ted) Ding and I am a PhD candidate in EPSRC Centre for Doctoral Training in Robotics and Autonomous Systems (CDT-RAS) programme, hosted at the Edinburgh Centre for Robotics and jointly offered by Heriot-Watt University and the University of Edinburgh. I am co-supervised by Dr. Sen Wang (Imperial College London) and Emeritus Prof. Andrew Wallace (Heriot-Watt University). My research aims to improve vehicular visual perception in challenging foggy weather. I have broad interests in signal processing and computer vision, using techniques including optimisation and deep learning.
I have submitted my PhD thesis. Currently I am working as a quantitative analyst intern at Deutsche Bank in London.
I obtained my BEng (First Class Hons) in Electronics and Electrical Engineering from the University of Edinburgh in 2013, and MSc (Merit) in Communications and Signal Processing from Imperial College London in 2014. Before starting my PhD study in 2020, I worked as a senior design/development engineer at Renishaw plc, where I was actively involved in designing and developing signal processing algorithms for the Renishaw RUP1 ultrasonic probe which was successfully launched in 2021, Together with my ex-colleagues, I co-invented the following two patents.
- L. D. Hall, Y. Ding, "Method of Calibrating an Ultrasound Probe and Corresponding Inspection Apparatus and Method", EP3931526B1, filed 17 February 2020, granted and published 30 August 2023.
- D. J. Wilson, R. N. Hand, Y. Ding, "Ultrasound Method and Apparatus", WO2023052757A1, filed 28 September 2022, published 06 April 2023.
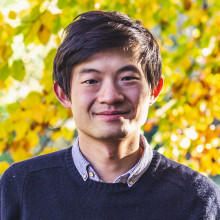