Dr Jose L. Part
Traditionally, robots have been confined to industrial settings where they operate in isolation and in highly controlled and structured environments to execute well-defined non-varying tasks. As a result, they usually operate without the need to perceive their surroundings or to adapt to changing stimuli. However, as robots start to move towards human-centred environments and share the physical space with people, there is an urgent need to endow them with the ability to learn and adapt given the changing nature of the stimuli they receive.
In this project, I will focus on the problem of lifelong object learning for robots operating in human-centred (dynamic and unstructured) environments. The main goal is the development of efficient and robust computational approaches to lifelong object learning. To this end, different types of models will be evaluated. Concretely, I will compare the use of growing self-organising networks to learn prototypes of the input data against the storage of real samples; the use of a simple nearest neighbour classifier against more complex classifiers; and I will also discuss the role that generative replay can play in more complex learning systems. Finally, I will develop a robot architecture to address lifelong object learning through situated interaction with a human partner. The situated nature of the interaction poses several challenges. For example, the system should be able to update its model in a reasonable time and learn efficiently so as to reduce the burden imposed to its human partner.
My current research interests include lifelong learning, situated interactive learning, hierarchical task learning, language grounding, learning through dialogue and visual demonstration, and cognitive systems. For a list of publications and more information, please visit my website!
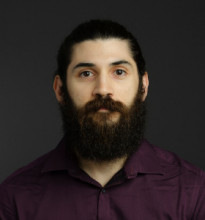