Konstantinos Gavrillidis
Explanation of autonomous behaviour is motivated by the need for transparency in critical applications and remote isolation that operators go through during mission supervision. The domain is autonomous underwater vehicles (AUVs) where transparency is even more critical due to the intermittent communication with the vehicle, deep underwater. Data-driven techniques will be explored that allow text-based interaction between the autonomy engine and the operator. Data2Text is a field of Natural Language Generation that typically takes one data source such as time-series data for weather, finance, biosignals. Here, we will investigate Data2Text deriving text explanations but from a mix of structured and unstructured data in various formats including log messages, a world model and dynamic environment, status from the vehicle, as well as, a representation of the autonomy model logic. The aforementioned data would be used as input to an end-to-end model that analyses these features and learns their relation and generates a natural description of the vehicle activity, status, mission success as well any errors or warnings. Investigating hybrid models such as hybrid code networks that can integrate the hand-written rules of the autonomy model as well as argumentation will also be investigated.
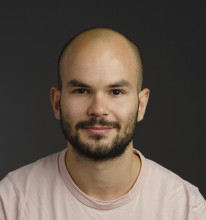