Remi Desmartin
Neural networks are increasingly relied upon as components of complex safety-critical systems such as autonomous vehicles. As a result, there is high demand for tools and methods that embed neural network verification in a larger verification cycle; the objective is to formally prove safety properties on trained networks, the main investigated property being robustness to noise in the data. This project aims to study the formulation and verification of properties that reflect the data's high level meaning beyond robustness; and the generation of checkable proofs to increase trust in the verification result. For that we use Imandra, a reasoning tool that combines aspects from interactive and automated theorem proving.
I graduated from Heriot-Watt in 2021 with a MSc Data Science degree. For my master's thesis, I worked on formalising fully-connected and convolutional neural networks in Imandra and verifying diverse properties on them, which won the Computer Science Prize for Best MSc Dissertation. My main research interest is neural network verification, i.e. how to guarantee the safety of neural networks in order to produce trustworthy autonomous systems.
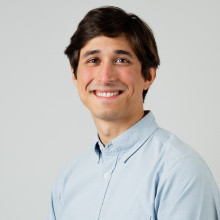